As a general purpose technology, artificial intelligence (AI) may be applied to many use cases. To structure potential application areas in the financial industry, a periodic table known from chemistry has been suggested (see Image below). The x-axis shows the abilities that determine intelligent behavior (AI) and the y-axis shows the complexity levels. It starts with applications that support the visual or acoustic perception of the world, e.g., for the recognition of images, speech or faces. In the case of visual perception, neuronal networks store characteristic features, such as eyes, nose and mouth, in order to recognize them as faces in other images. An example from the financial sector is the know-your-customer process (KYC), which supports the identification of “high risk individuals”. Areas of application with the functional scope of processing focus on the conclusive processing of the perceptions. Natural language processing (NLP) allows to recognize words with their meaning and, if the system is specified accordingly, also the mood of the text from the different word combinations, which might be valuable in oral or written conversations (sentiment analysis) with customers. Action comprises applications that involve actions to achieve specific goals.
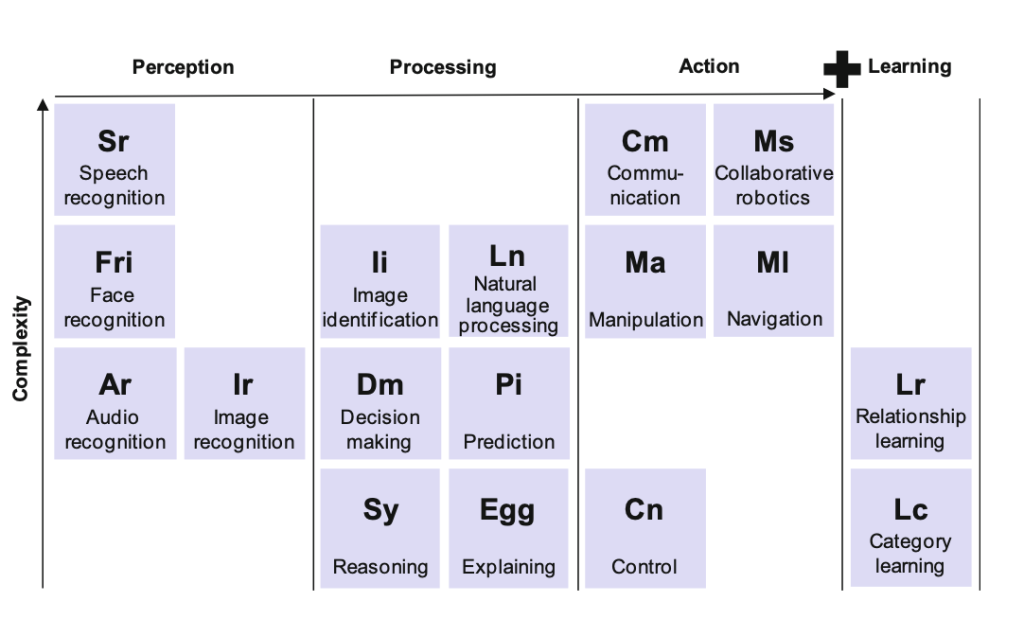
The simplest field is “control”, which refers to the control of software or hardware depending on defined measured variables. These could be automated AI systems for financial investments, which maximize the value of investment portfolios in predefined asset classes. If the AI system decides to buy or sell a security based on the preferences defined by the investor and the current market situation, it executes this transaction on an electronic exchange and adjusts the portfolio accordingly (robo advisory). In contrast, the field of application collaborative robotics is more complex, since (physical) robots are ultimately supposed to independently conduct actions that make sense in a given situation. An example is the (meanwhile discontinued) humanoid robot Pepper, developed by the Japanese technology company SoftBank Robotics for customer advisory services in bank branches. This robot was programmed to pre-select the products relevant to the customer while the customer was waiting for a conversation with the (real) customer advisor. The learning cluster enhances the areas of perception, processing and action. Intelligent agents may generate new knowledge about their environment during perception and processing as well as during the execution of actions. Category learning, for example, aims to learn combinations of characteristic values and thus to categorize them. Pepper, for instance, could categorize faces according to their mood (e.g., does the new visitor in a bank branch appear safe or unsafe because of his behavior?) and learn new subcategories from the category of “unsafe visitors” (e.g., visitors who appear to be looking for something and visitors who Pepper would classify as potential bank robbers). The application area of relationship learning goes beyond learning categories, in that the robot learns reactions to the respective category and assigns them to the categories (e.g., whether the customer is waiting for his appointment and might be happy about a first interaction with the expert robot). The robot could identify the faces of customers and analyze their social media contacts to determine other friends of the customer who have also recently visited the store. Customers in a corresponding network could receive individual benefits for referring friends. These responses could be supplemented by other interaction possibilities (e.g., Pepper could learn that he only has to show some visitors the way
dispenser if they are categorized to look uncomfortable in the summer), whereby the usefulness of such scenarios or their cost-benefit-risk ratio requires critical validation in each case.
More Term Definitions